PyTorch 实现一个 CNN 实例
以下示例展示如何用 PyTorch 构建一个简单的 CNN 模型,用于 MNIST 数据集的数字分类。
主要步骤:
- 数据加载与预处理:使用 torchvision 加载和预处理 MNIST 数据。
- 模型构建:定义卷积层、池化层和全连接层。
- 训练:通过损失函数和优化器进行模型训练。
- 评估:测试集上计算模型的准确率。
- 可视化:展示部分测试样本及其预测结果。
import torch import torch.nn as nn import torch.nn.functional as F import torch.optim as optim from torchvision import datasets, transforms
# 1. 数据加载与预处理 transform = transforms.Compose([ transforms.ToTensor(), # 转为张量 transforms.Normalize((0.5,), (0.5,)) # 归一化到 [-1, 1] ])
# 加载 MNIST 数据集 train_dataset = datasets.MNIST(root='./data', train=True, transform=transform, download=True) test_dataset = datasets.MNIST(root='./data', train=False, transform=transform, download=True) train_loader = torch.utils.data.DataLoader(dataset=train_dataset, batch_size=64, shuffle=True) test_loader = torch.utils.data.DataLoader(dataset=test_dataset, batch_size=64, shuffle=False)
# 2. 定义 CNN 模型 class SimpleCNN(nn.Module): def __init__(self): super(SimpleCNN, self).__init__() # 定义卷积层 self.conv1 = nn.Conv2d(1, 32, kernel_size=3, stride=1, padding=1) self.conv2 = nn.Conv2d(32, 64, kernel_size=3, stride=1, padding=1) # 定义全连接层 self.fc1 = nn.Linear(64 * 7 * 7, 128) # 展平后输入到全连接层 self.fc2 = nn.Linear(128, 10) # 10 个类别 def forward(self, x): x = F.relu(self.conv1(x)) # 第一层卷积 + ReLU x = F.max_pool2d(x, 2) # 最大池化 x = F.relu(self.conv2(x)) # 第二层卷积 + ReLU x = F.max_pool2d(x, 2) # 最大池化 x = x.view(-1, 64 * 7 * 7) # 展平 x = F.relu(self.fc1(x)) # 全连接层 + ReLU x = self.fc2(x) # 最后一层输出 return x # 创建模型实例 model = SimpleCNN()
# 3. 定义损失函数与优化器 criterion = nn.CrossEntropyLoss() # 多分类交叉熵损失 optimizer = optim.SGD(model.parameters(), lr=0.01, momentum=0.9)
# 4. 模型训练 num_epochs = 5 model.train() # 设置模型为训练模式 for epoch in range(num_epochs): total_loss = 0 for images, labels in train_loader: outputs = model(images) # 前向传播 loss = criterion(outputs, labels) # 计算损失 optimizer.zero_grad() # 清空梯度 loss.backward() # 反向传播 optimizer.step() # 更新参数 total_loss += loss.item() print(f"Epoch [{epoch+1}/{num_epochs}], Loss: {total_loss / len(train_loader):.4f}")
# 5. 模型测试 model.eval() # 设置模型为评估模式 correct = 0 total = 0 with torch.no_grad(): # 关闭梯度计算 for images, labels in test_loader: outputs = model(images) _, predicted = torch.max(outputs, 1) total += labels.size(0) correct += (predicted == labels).sum().item() accuracy = 100 * correct / total print(f"Test Accuracy: {accuracy:.2f}%")
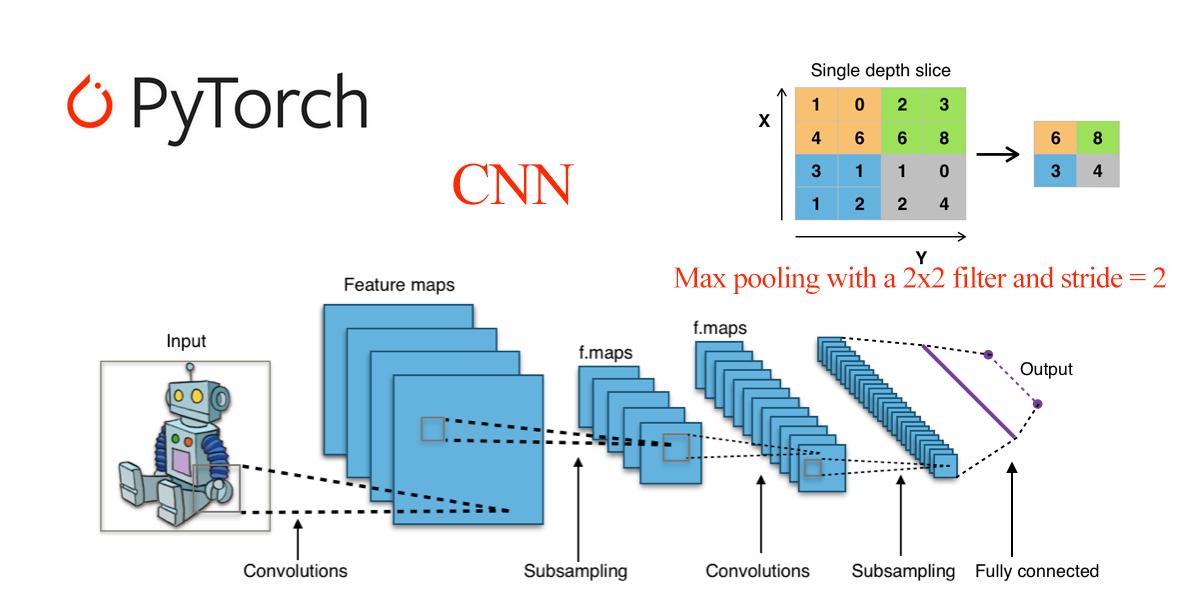